Must have AI Security Policies for Enterprises: A Detailed Guide
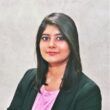
Last updated on: June 5, 2025
Introduction
In today’s rapidly evolving digital world, implementing a robust AI security policy is crucial to managing the growing risks associated with artificial intelligence. As AI becomes an integral part of businesses, it introduces unique vulnerabilities that demand attention. With remote work expanding the “attack surface” and the explosion of data from IoT devices and digital transformation, traditional security methods can no longer keep up. AI can enhance threat detection and response, but without proper policies, it can also become a target. Clear guidelines for AI security ensure that systems are deployed responsibly, risks are minimized, and compliance with industry regulations is maintained. Proactive planning is key to leveraging AI safely and effectively.
What is AI security, and Why is it Essential?
AI security is protecting AI systems and their data from risks and vulnerabilities. As organizations increasingly rely on AI, these systems become potential targets for adversarial attacks, fraud, and operational issues. Securing AI is essential to ensure its safe and effective use while minimizing risks.
- Detect Threats: AI can process massive amounts of data at lightning speed, identifying hidden threats that traditional security measures might overlook. This proactive approach helps mitigate risks before they cause significant harm.
- Prevent Fraud: By analyzing transaction patterns, AI can quickly identify suspicious activities, helping organizations combat fraud and protect financial systems effectively.
- Manage Vulnerabilities: AI can scan networks to uncover software flaws, outdated systems, or other weaknesses, allowing organizations to address and prioritize fixes before attackers exploit them.
- Enhance Endpoint Security: AI uses machine learning to detect unusual behavior on devices and block malicious activities, strengthening overall system protection.
- Automate Compliance: AI simplifies compliance by automating regulatory checks, generating accurate reports, and ensuring organizations meet legal and industry standards.
- Improve Physical Security: AI-powered video analytics can identify suspicious movements or activities in real-time, enhancing safety in physical spaces.
Key Threats to Enterprise AI Systems
AI has become one of the most powerful tools for businesses. However, it also introduces new security challenges. AI systems can be vulnerable to serious risks, compromising sensitive data, system integrity, and operational security without proper safeguards. Understanding these key threats is crucial to building a strong AI security framework and protecting against potential attacks.
1. Exposure of Sensitive Information
AI systems often process large volumes of data, some of which can include highly sensitive information like customer details, medical records, or financial data. If this data is not properly secured, it can be exposed during processing or storage, leading to data breaches that harm individuals and organizations.
2. Threats to AI Model Integrity
AI models can be manipulated or attacked in several ways:
- Adversarial Attacks: Hackers can intentionally alter inputs, like images or text, to deceive the AI and cause incorrect outputs.
- Model Inversion: Attackers can reverse-engineer AI models to extract sensitive data used during training.
- Data Poisoning: Attackers can corrupt the model and influence future decisions by introducing false or malicious data during training.
These attacks question the trustworthiness and reliability of AI systems.
3. Bias in AI and Regulatory Non-Compliance
AI algorithms can unintentionally develop biases if the data used to train them is unbalanced or flawed. This can lead to unfair outcomes. This includes discrimination in hiring or lending decisions. Moreover, regulatory frameworks demand transparency and fairness, making organizations need to address bias to avoid legal and reputational risks.
4. Operational Security Issues
AI systems are also vulnerable to operational challenges, including:
- Unauthorized Access: Without strict access controls, malicious actors can gain control of AI systems to steal data or disrupt operations.
- API Vulnerabilities: Many AI systems rely on APIs for communication, which can become weak points if not properly secured, allowing attackers to exploit them.
Must-Have AI Security Policies for Enterprises
As AI becomes an integral part of business operations, establishing strong security policies is essential to protect systems, data, and processes. A well-defined AI security policy ensures vulnerabilities are addressed, risks are minimized, and AI systems operate responsibly. Here are the key policies every enterprise should implement:
1. Risk Assessment
Regular risk assessments involve continuously scanning AI systems to identify vulnerabilities and potential threats. This proactive approach ensures that weaknesses, such as outdated software or misconfigurations, are addressed before they can be exploited. Frequent assessments help organizations stay ahead of evolving risks.
2. Data Security
Protecting data is crucial in AI systems. Policies should include encrypting data during storage and transmission, anonymizing sensitive information to safeguard privacy, and ensuring secure handling practices. These measures reduce the risk of breaches and ensure compliance with data protection regulations.
3. Access Control Policies
With Role-Based Access Control (RBAC), only authorized users can access AI systems based on their job roles. Adding Multi-Factor Authentication (MFA) further enhances security by requiring additional verification steps. These measures control unauthorized access and protect sensitive AI operations.
4. Incident Response Plan
An incident response plan prepares organizations for potential breaches or failures. It outlines detecting, containing, and resolving incidents while minimizing operational downtime. A clear strategy helps teams respond quickly and effectively, reducing the overall impact of a security event.
5. Transparency and Explainability
AI systems must be transparent and interpretable to foster trust. Enterprises should ensure that AI models can explain their decisions and are auditable. This transparency improves accountability and helps meet regulatory standards for fairness and ethical AI use.
6. Regular Monitoring and Audits
Monitoring and audits are essential to ensure AI systems remain secure and functional. This includes tracking for anomalies, assessing system performance, and addressing vulnerabilities as they arise. Regular oversight keeps AI systems reliable and reduces long-term risks.
Compliance and Regulations
An AI governance framework can be defined as a structured set of guidelines and regulations that ensures AI is developed and used responsibly, ethically, and safely. It serves as a roadmap to guide how organizations adopt and manage AI systems while minimizing risks and ensuring they align with societal and organizational values.
The purpose of an AI governance framework is to:
- Uphold Values: It ensures AI aligns with core societal values, ethical principles, and human rights, preventing harm or misuse.
- Address Risks: By tackling issues like bias, misuse, and unintended consequences, it reduces risks associated with AI systems.
- Foster Trust: A strong governance framework builds trust by showing that AI systems are managed transparently and responsibly.
- Promote Innovation: It creates a balance between encouraging innovation and implementing safeguards to prevent harm.
- Ensure Accountability: AI systems are ultimately overseen by humans, making organizations accountable for how AI is used and its impact.
How AI Policies and Governance Frameworks Work Together
AI policies and governance frameworks, such as the NIST AI RMF and ISO AI standards, play a vital role in ensuring AI systems are used responsibly, safely, and ethically. They complement each other by guiding how to manage AI risks while integrating security and trust into an organization’s AI processes.
The NIST AI Risk Management Framework (RMF) focuses on helping organizations identify and manage the risks associated with AI. It emphasizes trustworthiness and ethics, ensuring that AI systems are secure, fair, and transparent. This framework is particularly useful for creating policies that address potential risks like bias, misuse, or unintended consequences.
On the other hand, ISO AI standards like ISO 42001 offer detailed guidelines for incorporating AI into an organization’s programs. These standards provide a structured approach to AI systems management, ensuring they are integrated securely and aligned with organizational goals. They also emphasize the importance of compliance with industry regulations and global best practices.
Core Elements of AI Governance Frameworks
AI governance frameworks are built on key principles for responsible and ethical use of AI. Here are the core elements:
- Transparency: Ensures AI systems are open and their decisions can be understood and explained.
- Accountability: Holds organizations and individuals responsible for the outcomes of AI systems.
- Data Governance: Promotes secure, accurate, and ethical handling of data used in AI systems.
- Fairness: Ensures AI systems provide unbiased outcomes and do not discriminate against any group.
- Ethics: Guides AI development and use to align with societal values and moral principles.
Accountability Structures in AI Governance Frameworks
Accountability is a key part of AI governance frameworks, ensuring that people and organizations are held responsible for developing and using AI systems. This means that the individuals and teams creating AI, such as researchers, developers, and companies, must ensure their systems are safe, fair, and free from biases.
They are responsible for designing AI with safeguards to prevent errors or misuse and regularly monitor its performance. Accountability structures also require clear policies to address any issues that arise, ensuring those in charge take ownership of the outcomes. These structures build trust and ensure that AI systems align with ethical and security standards.
Ethical Guidelines for Using AI
These ensure that AI systems are developed and used responsibly. These guidelines include:
- Privacy: Organizations should protect user data through anonymization to maintain trust.
- Fairness: AI should be designed to treat everyone equally, avoiding bias or discrimination.
- Transparency: AI systems should be transparent about their work and easy to explain.
- Accountability: A designated person should oversee each AI project to address any problems or mistakes.
- Training: Employees need training on AI ethics to work with AI in a responsible way.
- Community Benefit: AI should be used to positively impact the community.
- Data Protection: Data must be kept safe when introducing new AI technologies.
- Representative Training Data: AI models should be trained using fair and balanced data to prevent bias.
- AI Governance Council: A council can help ensure the organization stays aligned with safe and ethical AI practices.
Processes for Continuous Improvement
The following processes can be used for the continuous improvement of AI use:
- Robotic Process Automation (RPA): Use software robots to automate repetitive tasks, such as data entry and invoice processing. This improves accuracy, reduces costs, and allows employees to focus on more valuable tasks.
- Process Optimization: AI can identify inefficiencies and process deviations, improving measurement accuracy. For example, AI-powered anomaly detection in manufacturing can help reduce downtime.
- Customer Confidence: AI can analyze data from markets, sales, social media, surveys, and customer feedback to help businesses make well-informed decisions.
- Process Mining: Generative AI can simplify process mining, which traditionally involves extensive data collection, manual mapping, and analysis.
- Documenting Processes: This involves revising existing processes to improve efficiency, standardizing workflows, implementing automation, and documenting the results for better performance.
Processes for Risk Mitigation
Here are some processes for mitigating the risks of AI use:
- AI Model Risk Assessment: AI can help assess and predict risks more accurately than traditional methods, ensuring better decision-making.
- Enhanced Risk Identification: AI can spot patterns and anomalies in large amounts of data, helping to identify risks faster and more accurately.
- Predictive Analytics: By analyzing historical data and current trends, AI can forecast potential risks before they happen.
- Scenario Analysis: AI can run simulations to understand how different risk scenarios might impact business outcomes.
- Cybersecurity Risk Management: AI helps detect cybersecurity risks by monitoring network activity, finding vulnerabilities, and responding to threats in real time.
- Risk Monitoring: AI can continuously track data, predict future risks, and simulate different situations to stay ahead of potential issues.
- AI Governance: A governance framework helps ensure AI systems are used responsibly, maximizing benefits while minimizing harm.
Building a Cybersecurity Culture Around AI
Building a strong cybersecurity culture around AI involves creating clear steps to ensure AI systems are secure, accountable, and aligned with overall security goals. Here’s how to go about it:
- Define AI Use Cases: Start by identifying where and how AI is being used in your enterprise. This helps you understand the areas needing special security and risk management attention.
- Map Data Flow Needs: Once you know where AI is used, look at how data flows through the system—from input to output. Secure this data lifecycle to prevent unauthorized access or misuse.
- Establish Roles and Responsibilities: Assign clear roles for AI system management and data security. Ensure accountability by designating people who are responsible for monitoring and protecting AI systems.
- Create an AI Incident Response Protocol: Develop a plan for handling security incidents involving AI systems. This should include playbooks or step-by-step guides on how to respond to breaches or failures.
- Integrate with IT Policies and Governance: Finally, ensure that your AI security practices align with the broader enterprise IT policies and governance framework. This will ensure that all parts of your organization are working together to protect data and systems.
Best Practices
- Regularly Update Policies: Since AI technologies and threats are constantly evolving, keep your policies updated to stay ahead of risks.
- Adopt Zero Trust Principles: Trust nothing and verify everything. Always ensure that users and devices are authenticated before granting access to AI systems.
- Use Tools for Anomaly Detection and Model Scanning: Leverage tools to detect unusual behavior or vulnerabilities in AI models.
- Conduct AI Security Training for Staff: Make sure your team is well-informed about the risks of AI and how to handle AI security issues responsibly.
- Use Third-Party Audits: Regularly have external experts review your AI systems and security measures to ensure they are effective and up to date.
By following these steps and best practices, you can create a strong cybersecurity culture that protects your AI systems and helps minimize the risks of AI.
Conclusion
Creating robust AI security policies is essential for enterprises to safeguard their AI systems, ensure ethical usage, and minimize risks. By implementing risk assessment, data protection, accountability, and compliance guidelines, organizations can secure their AI systems and foster trust with stakeholders. Regular updates, transparent practices, and strong governance are key to navigating the ever changing landscape of AI security. These policies form the foundation of a secure and responsible AI-driven enterprise.
Qualys TotalAI revolutionizes AI security by addressing the unique challenges of AI and LLM workloads. It delivers unmatched visibility into Generative AI infrastructure, from GPUs to model-specific risks, all aligned with OWASP Top 10 for LLMs. Integrated into the powerful Qualys TruRisk Platform, TotalAI offers a unified solution combining vulnerability detection, compliance, and threat mitigation.
What sets TotalAI apart? It actively safeguards against PII exposures and ensures robust data security, identifying risks like unintentional data leakage or unauthorized access within AI systems. With AI-driven insights, it predicts and prevents threats, automates compliance, and optimizes security resources—empowering organizations to embrace AI with confidence!
CTA – Experience unparalleled security and visibility with Qualys. Start your free trial now!
FAQ
- How can Enterprises Enforce AI Security Policies?
Enterprises can enforce AI security policies by implementing clear guidelines, assigning responsible teams, conducting regular audits, and integrating security measures into the AI development lifecycle. Automation tools, monitoring systems, and continuous training also help ensure compliance and effective enforcement of security protocols.
- What Tools are Available to Secure AI Systems in Enterprises?
Tools like anomaly detection systems, intrusion detection platforms, vulnerability scanners, and automated compliance checkers help secure AI systems. Solutions like Qualys, SIEM (Security Information and Event Management) platforms, and data encryption tools ensure protection against data breaches, misuse, and other AI-related risks.
- What is the Importance of Governance Frameworks for AI Security?
AI governance frameworks ensure the responsible development and usage of AI systems. They set accountability, transparency, ethics, and compliance standards, reducing risks such as bias, misuse, or unintended consequences. These frameworks foster trust, protect user data, and promote sustainable, fair AI practices across enterprises.
- What is an AI Security Policy, and Why do Enterprises Need it?
An AI security policy outlines the rules, processes, and tools for securing AI systems. It defines accountability, ensures data protection, and mitigates risks associated with AI use. Enterprises need it to manage potential threats, stay compliant, and build trust in AI while safeguarding sensitive data and operations.