AI Workload Security
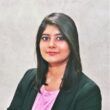
Last updated on: June 5, 2025
What is AI Workload Security?
AI workload security focuses on protecting the various tasks and processes that are carried out by AI systems within an organization. As AI gets increasingly integrated into business operations, ensuring the security of these workloads is crucial to maintaining the integrity of data and applications. Workload security involves safeguarding the computing resources that support AI models, ensuring they’re protected from threats, vulnerabilities, or misuse. This includes everything from the data being processed by the AI to the underlying infrastructure supporting it. By securing AI workloads, businesses can ensure that their AI-driven systems operate safely and reliably, meeting the necessary privacy and security standards while reducing potential risks.
What Are AI Workloads?
AI workloads are the tasks and processes in building, training, and using artificial intelligence systems. These tasks include preparing data, training models, and applying them in real-world situations. Since AI workloads are resource-heavy, they need much computing power, memory, and storage to efficiently handle large datasets and complex algorithms. Given their importance and demand for resources, it’s essential to have proper workload security and protection in place. This ensures AI workloads are secure from threats, providing smooth and safe operation of AI models across an organization’s systems.
Key Security Challenges in AI Workloads
Securing AI workloads has become crucial as AI becomes increasingly integrated into business operations. These workloads involve complex tasks and sensitive data, making robust security measures essential.
- High Computational Demands
AI workloads are resource-intensive, requiring significant computing power, memory, and storage. The infrastructure to support these workloads must be robust and secure to prevent potential vulnerabilities. Without sufficient protection, hackers can exploit weaknesses in hardware or cloud resources to gain unauthorized access to data or disrupt operations. - Data Privacy and Security
AI workloads often involve processing vast amounts of sensitive or personal data, making data privacy a critical concern. Organizations must ensure that data is protected both during processing and at rest. Proper encryption, access controls, and secure data storage are necessary to prevent breaches and meet regulatory requirements such as GDPR or CCPA. - Managing Large and Complex Datasets
AI systems rely on large, diverse datasets that can be difficult to manage and secure. Data quality and integrity are vital for accurate model training and effective decision-making. Without the right tools to monitor and secure data, AI workloads may inadvertently process faulty or malicious data, which could compromise the integrity of AI outputs. - Integration with Existing Systems
Integrating AI solutions into existing infrastructure can be challenging, especially when dealing with legacy systems that may lack the security features needed to support AI workloads. Organizations must ensure their AI models and underlying infrastructure are compatible and secure, requiring careful planning and expertise. - Ethical and Bias Concerns
AI algorithms are only as good as the data they are trained on, and bias in training data can lead to biased outcomes. Ensuring fairness and accountability in AI models is critical. Organizations must implement measures to identify and mitigate bias in AI algorithms to maintain ethical standards and trust.
Addressing these challenges requires a strategic approach to workload security and protection, coupled with the right infrastructure, tools, and skilled personnel, to ensure AI workloads are safe, efficient, and compliant.
Importance of AI Workload Security
AI workloads are transforming industries by enabling organizations to make faster, smarter decisions and innovate in once unimaginable ways. However, securing them becomes a top priority as these workloads handle large datasets, automate processes, and drive key business functions. Without proper workload security, businesses open themselves up to risks that can undermine the benefits of AI. Here’s why AI workload security is so important:
- Protecting Valuable Data
AI workloads often process vast amounts of sensitive data, from customer information to financial records. Ensuring this data is secure and protected from cyber threats is essential to maintaining trust and complying with privacy regulations. Without strong workload protection, data breaches could result in significant financial and reputational damage. - Ensuring Accurate and Reliable Insights
AI systems analyze data to make decisions and predictions that drive business strategies. If these workloads are compromised, the accuracy of the insights they generate can be undermined, leading to poor decision-making. Ensuring workload security helps maintain the data’s integrity, ensuring businesses make decisions based on accurate information. - Avoiding Disruptions and Downtime
AI workloads are often critical to business operations. If these systems are disrupted, it can result in downtime, decreased productivity, and lost revenue. Securing AI workloads helps prevent malicious attacks or errors that could bring these essential systems to a halt, ensuring smooth, uninterrupted operations. - Maintaining Customer Trust
AI workloads often deliver personalized customer experiences, such as through chatbots or recommendation systems. If these workloads are not adequately secured, it could compromise customer data or lead to poor service. Maintaining strong workload security is key to keeping customers’ trust and satisfaction. - Supporting Innovation Without Compromise
AI can drive innovation by automating processes, offering predictive analytics, and developing new business models. However, this innovation must be supported by strong security to prevent exploitation or misuse of the AI system. Workload protection ensures that AI remains a tool for progress without exposing the organization to unnecessary risks. - Scalability Without Sacrificing Security
As businesses scale their AI solutions, workload security must scale as well. Cloud-based AI services often offer flexibility, which can introduce new security risks. Ensuring that workload protection is in place at every level ensures that AI systems can grow without compromising security or performance.
The importance of AI workload security cannot be overstated. It safeguards AI systems’ data, integrity, and performance, ensuring businesses can leverage AI to its fullest potential while protecting themselves from risks.
Best Practices for Securing AI Workloads
Securing AI workloads is crucial to ensuring that the data and processes involved are protected from potential threats. As AI systems handle massive amounts of data and are integral to decision-making, having strong security measures in place helps maintain privacy, integrity, and trust. Here are some best practices to secure your AI workloads and ensure they function smoothly:
- Define and Maintain Data Boundaries
Defining and maintaining data boundaries clearly is essential to prevent unauthorized access. AI workloads should only use data appropriate for their access level. By establishing these boundaries, you ensure that sensitive data is only accessible to those who need it, reducing the risk of data leaks or misuse. - Implement Strict Data Access Controls
Controlling who has access to what data is a key element of workload security. Strict data access controls ensure that only authorized users and systems can interact with the AI workload’s data. This protects against unauthorized access or accidental exposure, helping maintain your data’s confidentiality and integrity. - Maintain a Data Catalog
A well-organized data catalogue helps track where all your data resides and who can access it. This makes it easier to manage and secure the data used by AI workloads. A data catalogue simplifies compliance efforts, ensuring data handling meets privacy regulations and security standards. - Create a Data Sensitivity Change Management Plan
Data can become more or less sensitive over time, so it’s essential to have a plan in place to handle these changes. A data sensitivity change management plan ensures that as data becomes more or less sensitive, the appropriate access controls and protection measures are updated. This proactive approach helps maintain data security throughout its lifecycle. - Secure AI Artifacts
AI models, training data, and algorithms are valuable assets, often called AI artefacts. Securing these artefacts ensures they are protected from tampering or unauthorized use. Implementing encryption and access restrictions for AI artefacts prevents them from being exploited or altered in harmful ways. - Safeguard Sensitive Data
Lastly, safeguarding sensitive data is a top priority in any AI workload. AI systems often process personal, financial, or proprietary data that must be securely stored and transmitted. Strong encryption, secure storage solutions, and regular audits help protect sensitive data from breaches and ensure it remains private and secure.
By following these best practices, organizations can effectively protect their AI workloads, ensuring they continue operating securely and efficiently while safeguarding valuable data. Proper workload security and protection are essential for building trust, maintaining privacy, and optimizing the performance of AI systems.
Addressing Potential Vulnerabilities in AI Workloads
AI workloads bring innovation and introduce security vulnerabilities that organizations must address to safeguard data and systems. By proactively mitigating these risks, businesses can ensure robust workload security and protect sensitive information.
Key Vulnerabilities and How to Address Them
- Data Security Risks
Protect data integrity, confidentiality, and availability throughout the AI lifecycle, from development to deployment, using encryption, access controls, and continuous monitoring. - AI Model Security Risks
Secure AI models’ architecture, weights, and parameters with strong access policies and model encryption to prevent theft, tampering, or unauthorized manipulation. - Adversarial Attacks
Safeguard against adversarial inputs and prompt injections by testing AI algorithms for vulnerabilities and deploying robust validation processes to prevent manipulation. - Ethical and Safe Deployment
When implementing AI systems, focus on fairness, transparency, and accountability to avoid biases, privacy violations, or false positives. - Regulatory Compliance
Ensure compliance with GDPR, CCPA, and the EU AI Act to protect sensitive data and avoid legal penalties. - Input Manipulation Attacks
Validating input data and implementing secure training processes to maintain accurate outputs can mitigate risks from input manipulation and data poisoning. - Supply Chain Attacks
Audit third-party components and software libraries used in AI development regularly to detect and address vulnerabilities that could lead to breaches. - AI Model Drift and Decay
Continuously monitor AI models for performance changes, retraining them when necessary to prevent adversaries from exploiting weaknesses.
Addressing these vulnerabilities can strengthen workload security, ensuring organizations’ AI systems remain resilient and reliable.
The Role of Governance and Compliance in AI Workload Security
As organizations increasingly adopt AI workloads, governance and compliance are vital in ensuring AI systems’ security, integrity, and ethical use. By establishing clear policies and adhering to regulatory standards, businesses can mitigate risks and build trust in their AI-driven operations. Here’s how governance and compliance contribute to workload security:
- Defining Clear Security Policies
Governance frameworks set the foundation for AI workload security by establishing clear data access, processing, and storage policies. These policies help ensure that AI systems operate within defined security parameters and prevent unauthorized access to sensitive data. - Adhering to Legal Regulations
Compliance with regulations such as GDPR, CCPA, and the EU AI Act is crucial for protecting data privacy and ensuring lawful AI usage. Adhering to these laws reduces the risk of data breaches and legal penalties, safeguarding both organizations and end-users. - Ensuring Ethical AI Practices
Governance ensures that AI workloads are developed and deployed ethically, avoiding biases, unfair treatment, or unintended consequences. This fosters transparency, accountability, and fairness in AI decision-making. - Monitoring AI Lifecycle Risks
Effective governance includes regular audits and monitoring of AI workloads throughout their lifecycle. This proactive approach helps identify and address risks, such as model drift or data vulnerabilities, ensuring consistent workload security. - Third-Party Risk Management
Compliance frameworks guide organizations in managing risks associated with third-party vendors and components used in AI development. Regular evaluations and contractual safeguards help mitigate vulnerabilities in the supply chain. - Building Organizational Trust
Strong governance reassures stakeholders, clients, and regulators that the organization takes security and ethical considerations seriously. This trust enhances the organization’s reputation and allows wider AI adoption.
By integrating governance and compliance into AI workload security strategies, organizations can protect their systems, comply with laws, and uphold ethical standards in their AI operations.